When handling sensitive medications or biological samples, robotic automation solutions must embody one particular human-like ability: accurate perception of their surroundings. The use of stereo vision in AMR robots ensures the accuracy of item and material identification for laboratory and healthcare environments.
Misidentification of a medication or accidental contact with unsterilized equipment can trigger costly delays or far worse consequences. Stereo vision technology empowers autonomous mobile robots (AMRs) to take on larger roles in Life Sciences and Healthcare via the ability to perceive depth, similar to their human coworkers.
Companies like Quasi Robotics refine the stereo machine vision abilities of their autonomous robots for better navigation and safety, resulting in sophisticated solutions that are well-suited for the demands of laboratory and healthcare settings.
Table of Contents
What is Stereo Vision
The foundation of stereo vision lies in replicating the way our eyes perceive depth. We see the world from slightly different angles with each eye, and our brains process those differences to determine how far or near objects are.
Just as humans have two eyes, each with slightly different perspectives, a stereo vision camera uses two cameras to capture images. By analyzing the differences between these images, the robot’s onboard computer can calculate its distance to objects in its environment.
Stereoscope Definition
The concept of the stereoscope harnessed this principle in the 1800s. The stereoscope definition refers to a device that uses two, nearly identical, images viewed through separate lenses, to create a 3D illusion. Modern stereoscopic vision takes this a step further by utilizing camera stereo vision – two digital cameras working in tandem to generate a real-time depth map of the environment.
- Precise Calculation: Accuracy is achieved through careful calibration, ensuring the cameras are perfectly aligned and their positions are known, minimizing errors in depth calculations.
- Triangulation: A stereo vision camera uses a technique called triangulation. By knowing the distance between the cameras (the baseline) and the angle of each camera relative to an object, its distance can be calculated.
- Computational Power: The calculations involved in stereo vision are complex. Modern AMRs have onboard computers powerful enough to process camera images in real-time, constantly updating their understanding of the environment.
Active vs. Passive Stereo Vision
Stereo vision systems come in two main varieties: passive and active. The primary difference lies in how they acquire visual information about their surroundings.
- Passive Stereo Vision: Relies solely on ambient light in the environment to capture images. This approach is often preferred due to its lower power consumption and greater simplicity.
- Active Stereo Vision: Employs a structured light source, such as a projector, to illuminate the scene. This can improve accuracy in low-light conditions or when dealing with objects with few visual features.
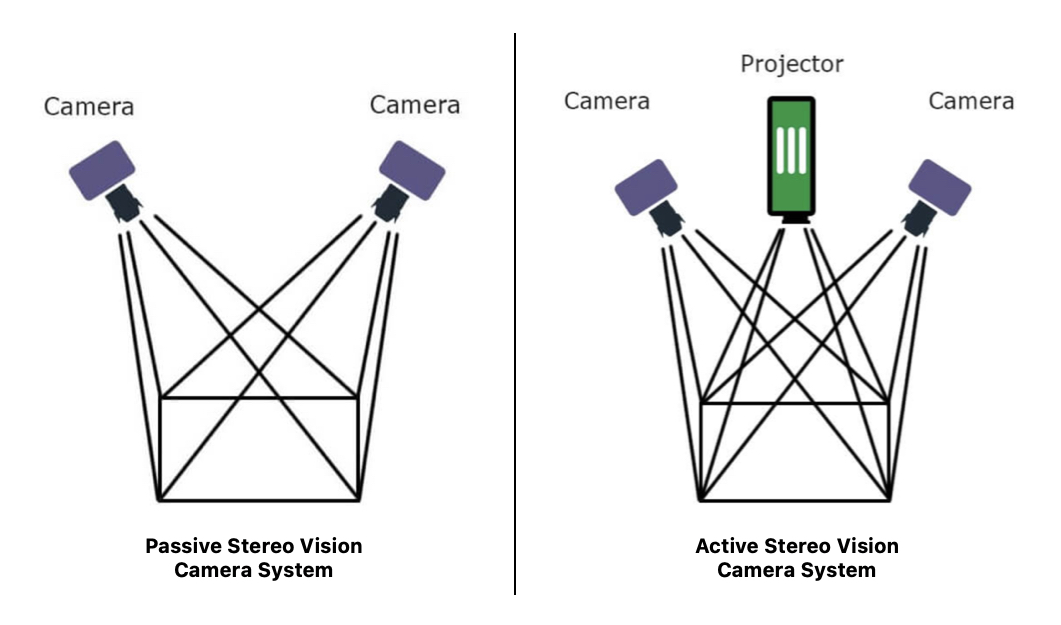
The Role of Stereo Vision in AMR Success
Stereo vision equips AMRs with the ability to perceive their surroundings in 3D. This capability is essential in healthcare and laboratory settings, where precise object identification, spatial awareness, and safe navigation are critical for successful operation.
Object Recognition & Detection
For AMRs operating in healthcare and laboratory environments, the ability to accurately identify objects is critical. Stereoscopic vision provides AMRs with an accurate understanding of objects in their workspace. By comparing the images from two stereo vision cameras, the system can identify and classify objects based on their shape, size, and texture.
Autonomous mobile robots leverage stereo computer vision In practical settings healthcare and laboratory settings, where robots may need to distinguish between various medications, lab instruments, or biological samples & delicate specimens. Ensuring accuracy in this task is paramount for patient safety and to maintain the integrity of research.
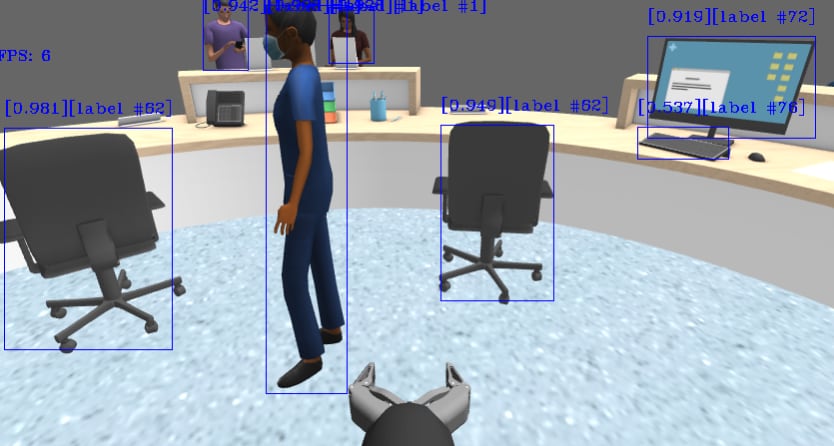
Area Mapping & Localization
Stereo vision plays a vital role in an AMR’s ability to navigate its environment. By generating a detailed 3D map of the workspace, a stereo camera robot can understand its position within that map. As the robot moves, it analyzes the environment from different angles, allowing it to locate itself and understand obstacles or changes in its path.
Stereo computer vision allows robots to adjust their routes in real-time, detecting changes and unexpected obstacles, and ensuring efficient navigation and safe operation, even in dynamic environments.
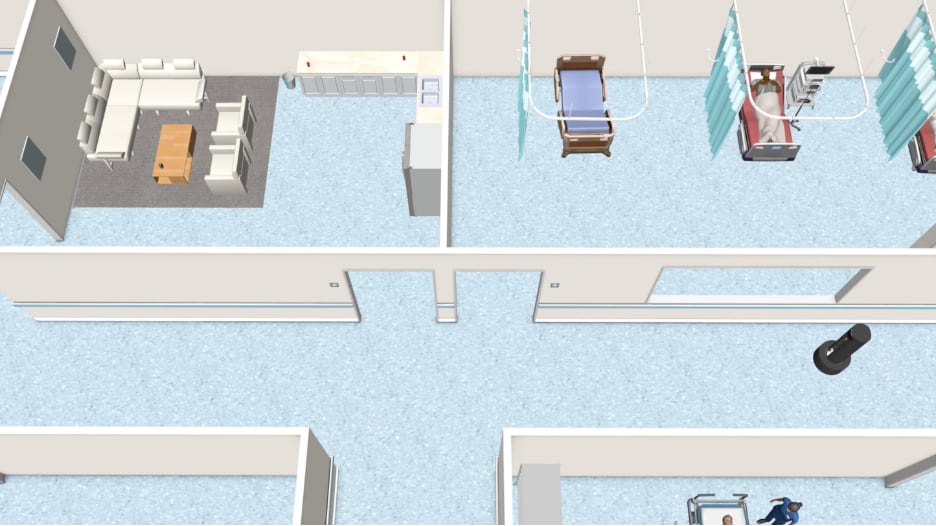
More Advanced Stereo Vision Uses
Precision Manipulation
By accurately understanding the 3D shapes and positions of objects, robots could execute delicate tasks that currently require human intervention.
- Consider the scenario of a robot stereo vision camera safely guiding a robot arm to transfer fragile biological samples between containers or assisting a surgeon by manipulating tools during a minimally invasive procedure – the possibilities are significant.
- This capability would be a game-changer, particularly in settings where precision and sterility are paramount.
Stereo Computer Vision Integration for Augmented Reality (AR)
The marriage of AR technology with stereoscopic vision example systems could greatly enhance situational awareness for technicians in healthcare and laboratory environments. A lab technician could use stereo vision to see vital information overlaid on their view of an AMR via an AR headset as the robot performs a delivery.
- This info could include real-time navigation data, the contents of its payload, or any safety alerts relevant to the robot’s surroundings.
- AR-integrated camera stereo vision could also facilitate remote monitoring and operation of robots, streamlining workflows across geographically dispersed labs.
Collaborative Workspaces
As robots and humans increasingly share workspaces, binocular stereoscopic vision could play a crucial role in safe and efficient human-robot collaboration. By constantly assessing its surroundings in 3D, a robot can detect the presence and movements of humans, proactively adjusting its path or speed to prevent collisions.
- This technology paves the way for seamless teamwork between humans and robots, allowing them to safely focus on increasingly complex and specialized tasks.
Real-World Applications of Stereo Vision in AMR Solutions
To better illustrate the practical applications of stereo vision in healthcare and labs, let’s consider a few specific examples where object identification is critical:
- Medication Identification: Distinguishing between vials of different medications based on their shape, size, and printed labels prevents errors and ensures accurate delivery, particularly crucial in critical care settings.
- Surgical Tool Tracking and Inventory: Identifying surgical instruments (scalpels, forceps, etc.) streamlines tool preparation and sterilization processes. The robot ensures the right tools are always available, reducing downtime and minimizing infection risks.
- Hazardous Material Recognition: Identifying hazardous material containers based on markings, shape, or color enhances safety. The robot can alert personnel of dangerous substances or transport them with extra precaution, reducing the risk of spills or contamination.
Challenges of Stereo Vision & Potential Solutions
While stereo vision unlocks remarkable capabilities, it’s important to acknowledge potential challenges and the ongoing innovations designed to surmount them. Two common difficulties include:
Lighting Variations: Hospitals and labs can have inconsistent or harsh lighting (bright surgical lights, dimmed storage areas). These variations pose a challenge. Solutions include:
- Employing advanced algorithms that adapt to different lighting conditions.
- Integrating ambient light sensors with the stereo vision system to enable dynamic adjustments.
Reflections: Shiny surfaces like metallic instruments or glass vials can create reflections that confuse traditional image analysis. Solutions include:
- Using polarization filtering techniques to reduce glare.
- Employing specialized image processing software that can overcome reflections and maintain accuracy.
Case Study: Stereo Vision in AMRs from Quasi Robotics
Quasi Robotics’ Model C2 and Model R2 AMR robots expertly demonstrate how stereo vision translates into real-world benefits for healthcare and laboratory environments. It practical applications, along with the technical foundations behind them, include the following.
- Accurate Inventory Tracking: Model R2 determines the orientation of boxes and vials and verifies their labels to accurately locate items during inventory. This reduces errors and minimizes the need for manual re-checks, streamlining quality control and stock reordering.
- Sterile Zone Navigation: Model C2’s robot stereo vision camera enables it to differentiate between designated sterile and non-sterile zones within a lab. This helps adhere to equipment integrity protocols and improves workflow efficiency within critical environments.
- Precise Obstacle Detection: The C2 robot stereo vision camera detects unexpected obstacles, like carts or other equipment, to avoid collisions and create safer workspaces.
- Enhanced Object Manipulation: Through continued refinement of the stereoscope definition, future robots may identify optimal grasp points for objects and calculate perfect dexterity for sensitive materials.
Lab & Healthcare Pain-Points Addressed by Stereo Vision
- Maximizing Uptime: Stereo vision’s precise navigation and obstacle detection reduce accidents and collisions. This minimizes unexpected downtime, keeping robots operational and workflows uninterrupted – crucial in time-sensitive healthcare and research environments.
- Enhancing Safety Protocols: Accurate depth perception enables safe human-robot interaction. When robots can identify and avoid workers, delicate equipment, and unforeseen obstacles, it safeguards both people and valuable assets, minimizing risks in shared workspaces.
- Reducing Errors: Stereo vision allows reliable identification of medication, inventory items, and critical equipment. This streamlines workflows, minimizes human errors, and ensures data integrity – a major concern in labs and healthcare.
Q.AI Stereo Vision: The Quasi Software Advantage
Quasi Robotics goes beyond merely integrating stereo vision hardware. Our in-house software development team crafts specialized algorithms and image processing pipelines that extract maximum value from the camera systems and enhance robot capabilities. Here’s a glimpse into those software elements:
- Calibration and Refinement: Quasi’s custom calibration procedure ensures cameras are aligned and working in tandem. Software fine-tunes the system over time, adapting to wear and tear to maintain maximum accuracy for real-world use.
- Object Detection and Classification: Proprietary algorithms analyze the stereo image data to reliably detect objects of various sizes, shapes, and textures, with software tailored for identifying lab and healthcare equipment, vials, etc.
- Mapping and Localization: Specialized software modules build detailed 3D maps from stereo vision data, allowing Quasi robots to understand their environment. These maps are updated in real-time, ensuring adaptability to any changes within the workspace.
- Optimized for ROS: Ensures smooth data flow, enabling AMRs to use depth information for path planning, obstacle avoidance, and precise navigation.
The Result: This emphasis on software development gives Quasi Robotics robots a distinct edge. Our stereo vision systems are more than a feature –– they’re deeply integrated into our AMRs’ intelligence, enabling them to go beyond basic functionality and tackle the nuanced challenges of healthcare and laboratory environments.
The Future Potential of Stereo Vision in AMR Success
Synergy of Machine Learning & Stereo Vision in AMRs
A key focus area for innovation is the integration of stereo vision with machine learning/AI. This combination has the potential to unlock even greater precision and adaptability in the following areas:
- Maximizing Uptime: Stereo vision’s precise navigation and obstacle detection reduce accidents and collisions. This minimizes unexpected downtime, keeping robots operational and workflows uninterrupted – crucial in time-sensitive healthcare and research environments.
- Object Recognition in Unstructured Environments: AI-powered image analysis can train stereo vision systems to detect items even in cluttered or partially obscured situations. This is especially beneficial in busy labs or healthcare facilities where conditions aren’t always pristine.
- Adaptive Decision-Making: Machine learning models can analyze stereo vision data in real-time to enable more complex decision-making for the AMR. For instance, the robot could learn to identify areas with higher foot traffic and proactively adjust its route to minimize disruption.
Maximizing Stereo Vision Potential in AMRs
The future of stereo vision for AMRs emphasizes advancements that will allow robots to navigate more effectively and safely within complex and ever-changing environments. Key research areas seek to refine how these systems process information and adapt over time. Here’s a look at some developments that hold the potential to significantly enhance AMR capabilities.
Enhanced Obstacle Detection & Mapping
Research is focused on improving algorithms for real-time object recognition, even within complex and dynamic environments typical of hospitals and labs. This would enable more nuanced navigation and obstacle avoidance.
Advanced Sensor Fusion with Stereo Cameras
Researchers are exploring the combination of stereo vision data with other sensors like LiDAR to create even more comprehensive 3D representations of a robot’s surroundings. This could further enhance navigation and safety.
Collaborative Human-Robot Workspaces
Emphasis is placed on developing stereo vision systems that can accurately track and predict human movements. This would facilitate seamless and safe collaboration between humans and AMRs in shared workspaces.
Self-Calibrating & Adaptive Systems
Future stereo vision systems could potentially incorporate feedback mechanisms for self-calibration and adaptation. This could involve:
- Adjusting for Environmental Changes: Automatic compensation for changes in lighting or appearance of new objects in workspace, maintaining accuracy without manual intervention.
- Continuous Learning for Optimization: Learning from experience via combination of feedback and sensor data to refine depth perception accuracy and improve navigation & object detection.
Conclusion on Stereo Vision in AMRs
From precision object recognition and enhanced safety protocols to the groundbreaking potential for tasks like micro-manipulation, stereo vision gives robots a crucial understanding of their environment. This understanding enables reliable navigation, adaptability to complex spaces, and opens a path for safe and efficient human-robot collaboration in the future.
Discover how Quasi Robotics leverages advanced stereo vision systems to provide cutting-edge AMR solutions tailored for labs and healthcare.